Continuous Glucose Monitoring (CGM) devices are becoming more commonly spotted on the arms and shoulders of professional triathletes as interest has risen in the last couple of years. CGMs are made from a small sensor — not a needle but a filament with glucose-sensing enzymes— just under the skin with a processing chip and data transmitters in a protective plastic housing.
Many CGMs can connect to a smartphone, smartwatch or cycling head unit to transmit live data about your glucose levels from the interstitial fluid. By including context from additional factors, including exercise, mood and diet, we can inform decisions on food timing and types of nutrition to leverage for performance.
A CGM measures interstitial glucose, the amount found in the fluid between the cells. These values within the interstitial fluid are relative to glucose in the blood, which has a higher glucose content. Despite this indirect measurement, the relationship is modeled well enough with mathematics that blood glucose values can be calculated algorithmically from interstitial glucose.
A 2016 paper published in the National Library of Medicine entitled Modeling and Measurement of Correlation between Blood and Interstitial Glucose Changes highlights how the authors have developed a “compensatory tool for measuring the delay in ISF glucose variations in reference to blood glucose changes.” Much of the industry has not had a chance to incorporate the mathematical work done in academia into their CGM models with relatively recent research.
The paper states, “Several factors cause concentration differences and a time-lag between blood and ISF glucose levels, including different distributions of glucose between blood vessels and subcutaneous tissue, glucose permeability, blood flow, and changes in the release of pancreatic hormones.” The aspects of time lag revealed are particularly of note as glucose levels are changing quickly, including as prone to change athletes’ bodies are during endurance sports.
Broadly speaking, these algorithms work, and the mathematics involved aren’t particularly complicated. The Freestyle Libre CGM — the sensor I’ve been using while testing a sport-specific monitoring app — has an error of 9.7% over 14 days. Whereas finger prick tests tend to be in the range of 5-10%. The error rate of the Libre is slightly on the high side, but it’s approved for clinical use in the world of diabetes, meaning it’s good enough for athletes. You can certainly trust the current generation of glucose monitors. If you have a finger prick glucose monitor, it can cross reference the values shown on your athlete’s CGM.
Glucose 101
Glucose is one of the various substrates our body uses for energy. Completing a movement requires energy, and endurance sport requires multiple repeated movements. The amount of glucose available impacts performance levels. Yet, it’s not simply that more glucose is better. Rushes and troughs in blood glucose can lead to unpredictable moods and performance. And more importantly, when exposed to this high variability for a long time through unhealthy habits related to the lack of regular exercise, poor food choices and simply a mix of bad decisions related to these parameters, long-term health problems can occur. Coaches and athletes with diabetes will be more familiar with glucose and its monitoring, which they must manage carefully. For people with diabetes, it is harder to regulate their glucose levels effectively; thus, they must keep this stable.
Carbohydrate loading can help increase glucose levels before sessions to improve energy availability, which is associated with better performance in endurance activities. Food is not the only thing that can affect glucose but overall is internally regulated by insulin and glucagon secreted by the pancreas.
Lessons from Using a CGM
Recently, I’ve been using a CGM from Supersapiens and have learned several things. I find with any device, one must understand it in the context of themselves before applying it to other athletes. (editor’s note: Supersapiens has formally shut down their app and business, but other CGMs are available.)
NOTE: EXAMPLES BELOW ARE FROM SUPERSAPIENS DATA DASHBOARD (available to all users)
THE RED HORIZONTAL LINE IS CONNECTED TO THE EVENT THAT EACH EXAMPLE REPRESENTS
1. Pre- and post-dinner walks are rarely a bad idea
Activity levels immediately before and after eating can make a massive difference to the response in glucose levels from eating. I found that taking a walk immediately before and after a meal stabilized my glucose levels. This meant there was no significant spike or drop from meal times, and I avoided the post-meal sluggishness I often felt. It also told me I made more sensible food choices when I did sit down to eat. Through this visibility, I could make informed decisions about my food.
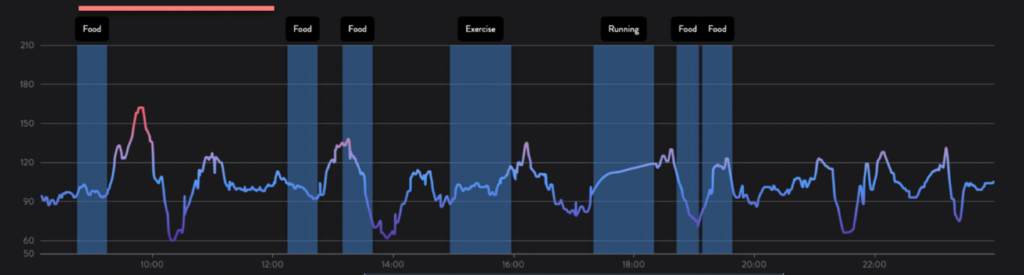
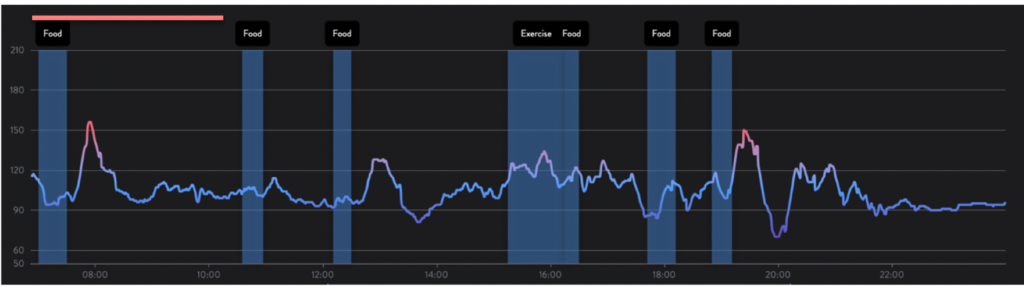
2. The order you eat your food matters
Carbohydrate increases glucose levels more than other food types, which makes intuitive sense. When you sit down to eat, consume your fat and protein first, followed by carbs. Any sudden rise in glucose is usually met with a corresponding crash in a “what goes up, must come down” effect. Avoiding the post-meal crash by eating your vegetables first is a good way of maintaining glucose stability with relatively little intervention.
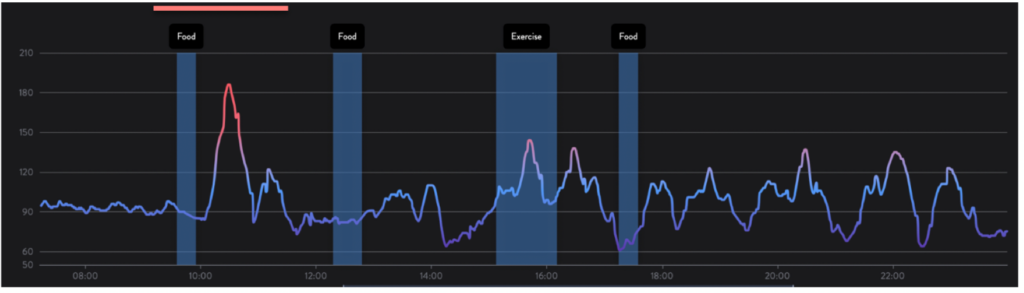
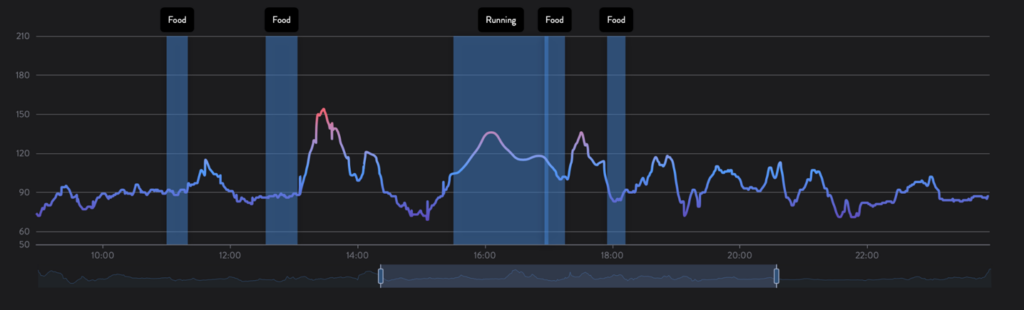
3. The data without knowledge is useless
A CGM will present you with a wealth of data. Every activity and action will be associated with a corresponding rise or fall in blood glucose, which can lead to an overwhelming volume of information. As with any data, enough domain knowledge to understand what it’s telling you is vital – without this, you may as well not collect the data in the first place. A concerted effort to keep a diary of food, mood and movement is required in order to make sense of this. The necessary data for helpful CGM is collected not just by the sensor but also by all the contextual information. It’s a sizable cognitive load that your athlete should be aware of before committing to using one.
4. Mood matters a lot
Food and exercise are not the only things that impact glucose response. Your body regulates glucose levels with hormones, and these hormones can be set off with all sorts of life events. Stress can elevate glucose, something many see as an evolutionary response. Increased glucose levels can improve one’s ability to engage in a ‘fight or flight’ as a response to a stressor.
5. Experiment with your food
You’ll notice that food timings, types and all sorts impact your glucose response and taking a scientific approach to this is vital to getting the most out of your CGM. Write everything down, many of the apps associated with the CGM allow you to do this, but if not, you can make use of the Training Peaks notes and integration. Alternatively, you and your athlete can simply share a cloud-based spreadsheet or document. Having a food diary is essential for making the most of the CGM, but care should be taken with athletes who have had a history of eating disorders.
Should I Tell My Athletes to Get One?
CGMs are not a necessity by any stretch. Your athletes can live without one. It’s definitely a sensor that I would put in the ‘nice to have’ pile, but it can be advantageous, provided you work to record and use the information generated. Just as in diabetic individuals, who have realized the benefits of CGMs much earlier than athletes, many athletes see improved glucose control from utilizing them. Still, once the lessons are learned, continued use of the CGM isn’t necessary. It’s probably most useful as an educational tool more than anything. Athletes can see the impact of their food choices very clearly.
Ultimately, it’s a device that will require extra work on behalf of the athlete—keeping a diary of their food, mood and non-training movement to cross-reference the actions causing spikes in glucose levels. If your athlete has the capacity and is willing to do this work, CGM can provide valuable information, but it’s not a stick-and-forget device—even if the coach analyses all the data. If you have a really busy athlete with no capacity for extra work, this device will be less useful. The usefulness of a CGM scales with the commitment one puts into cultivating high-quality information from it.
References
Shi, et al. 2016. Modeling and Measurement of Correlation between Blood and Interstitial Glucose Changes. Retrieved from https://www.ncbi.nlm.nih.gov/pmc/articles/PMC4863111/
Wilber, R., Moffatt, R. Influence of Carbohydrate Ingestion on Blood Glucose and Performance in Runners. Retrieved from https://journals.humankinetics.com/view/journals/ijsnem/2/4/article-p317.xml